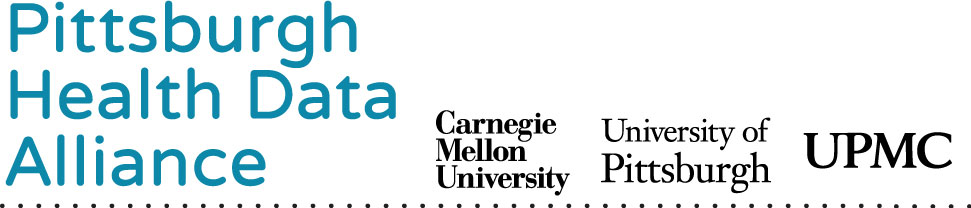
Three Pittsburgh Institutions. One Goal.
The Pittsburgh Health Data Alliance represents an unprecedented collaboration between Carnegie Mellon University, The University of Pittsburgh, and UPMC.
By combining forces, we have an unparalleled opportunity to move learning derived from data into solutions that can seamlessly reach patients. The University of Pittsburgh brings expertise in medical research, Carnegie Mellon University brings world-class computer science and machine learning, and UPMC brings deep data, the clinical setting, and a track record of successful commercialization.
Leadership
Carnegie Mellon University
Contact us by email.
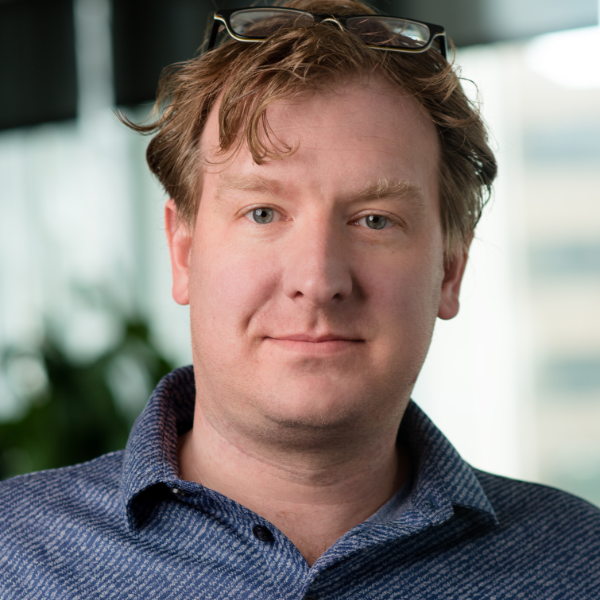
Carl Kingsford, PhD
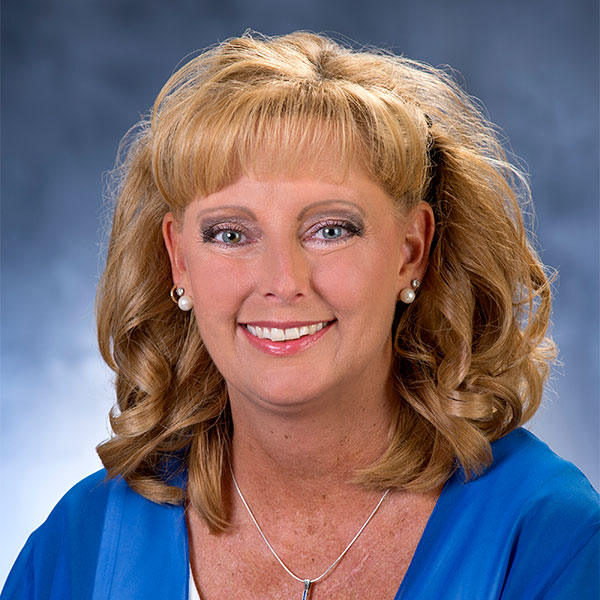
Heather Johnson
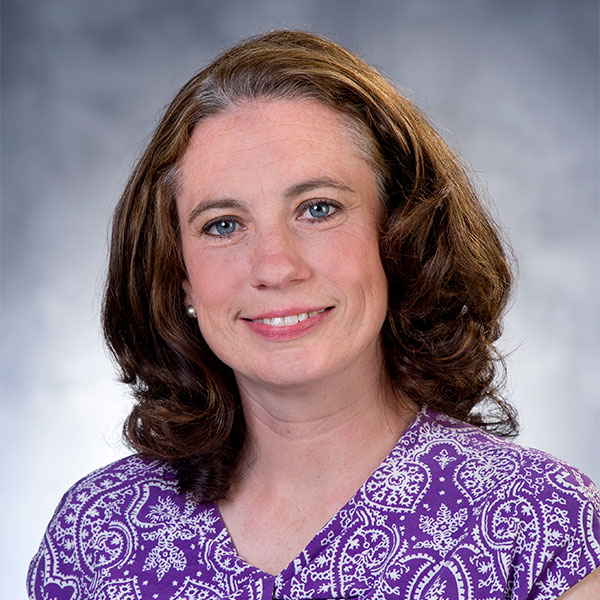
Nicole Flynn
University of Pittsburgh
Contact us by email.
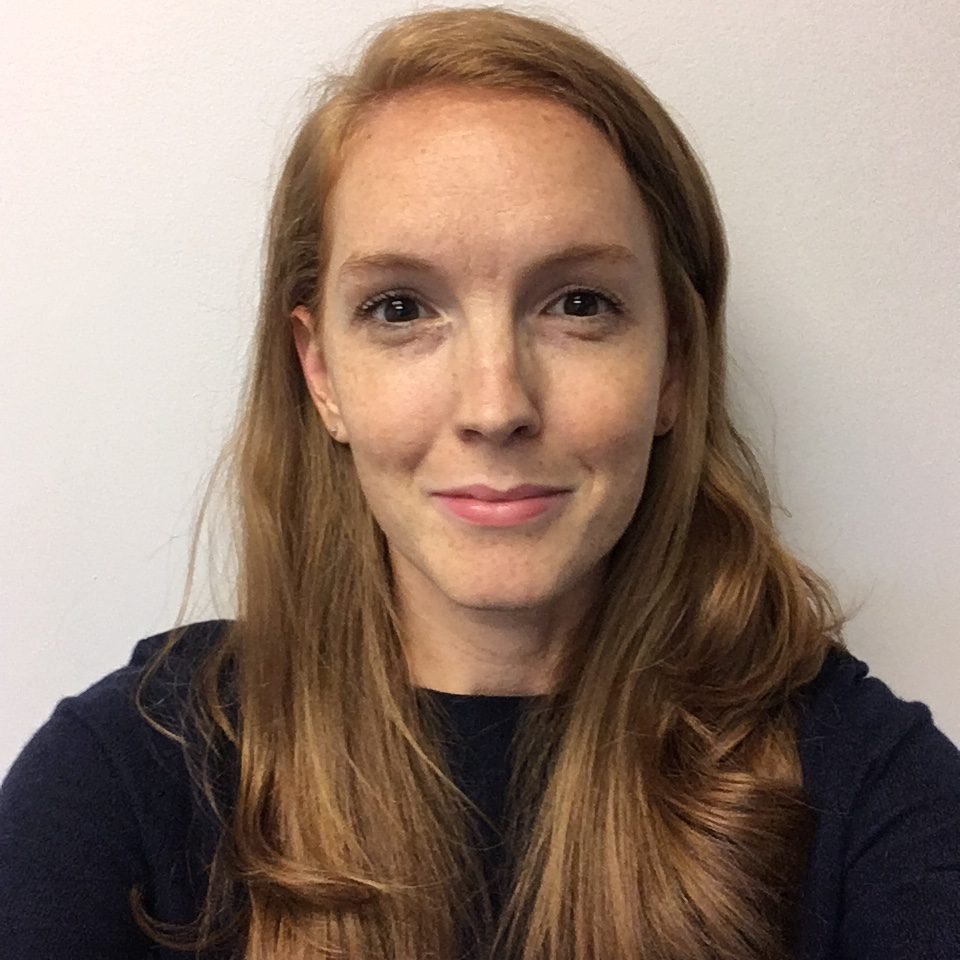
Colleen Cassidy, PhD
UPMC Enterprises
Contact us by email or visit the UPMC Enterprises contact form.
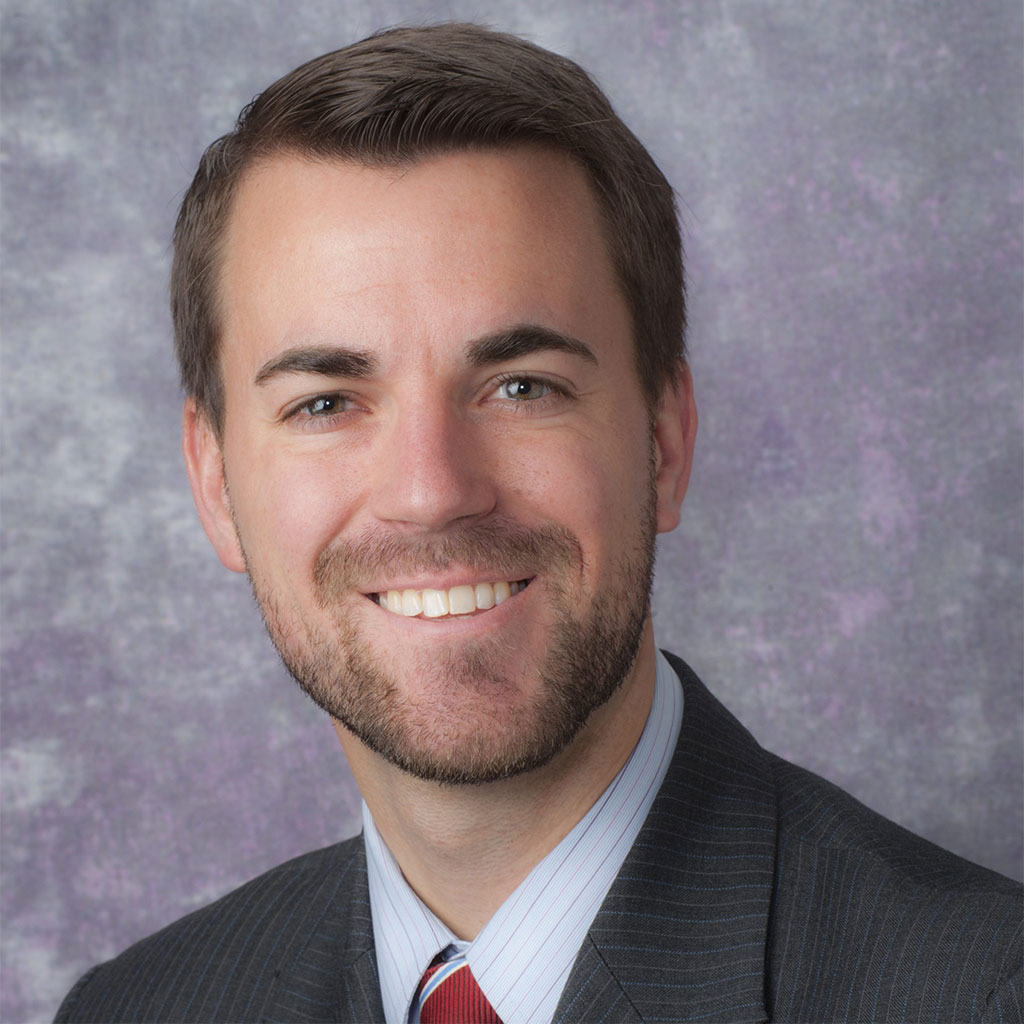
Chad Dehmer
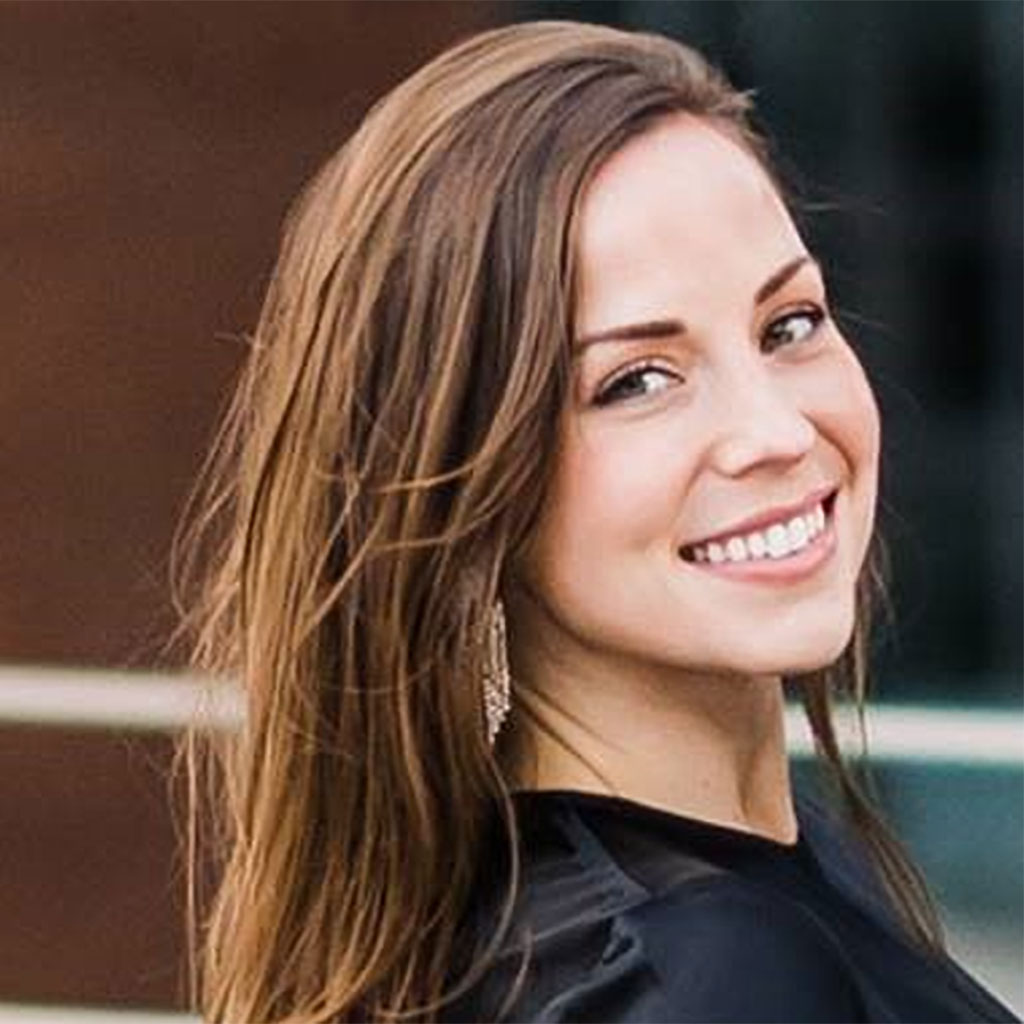
Dana Sommer